Can Data-Driven Approaches Really Improve Infertility Treatment Outcomes?
- Francois Smuts
- Feb 20, 2024
- 4 min read
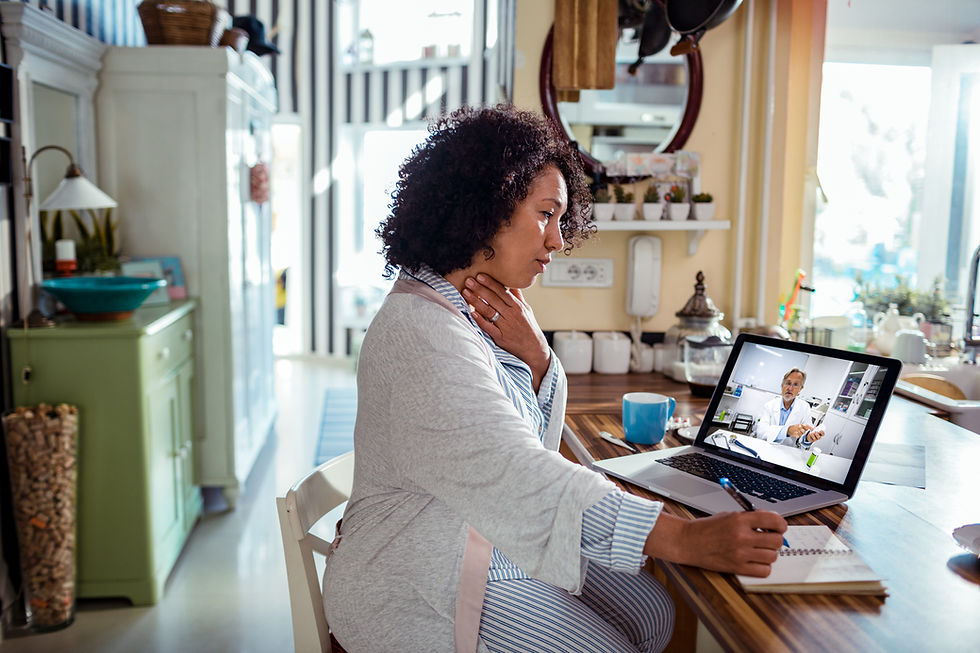
Infertility is a sensitive issue affecting millions worldwide. Once considered a private tragedy, new data-focused tools are shedding light on its underlying causes and connections in ways that empower both patients and doctors. Advanced data collection and machine learning algorithms now provide a comprehensive view of infertility, spotting patterns that illuminate new avenues for diagnosis and customized care.
This record revolution in reproductive medicinal drugs represents the dawn of a new technology. Where faith and prayer as soon fill the void of medical expertise, objective information now takes their location - guiding selections with scientific precision and providing many couples hope wherein none existed earlier.
This article will discover how modern facts-driven procedures are demystifying and destigmatizing infertility. We'll cope with the role of data in diagnosing root problems, the promise of synthetic intelligence in treatment-making plans, and moral considerations around new predictive technology. By shining a mild on the connections among lifestyle, genetics, and fertility, those answers empower people to play a lively function in overcoming demanding situations.

The Power of Aggregated Patient Data
Traditionally, fertility issues were assessed on an individual basis through clinical exams and testing. However, this narrow view provided an incomplete picture. Thanks to a large collection of anonymous patients, new systems have emerged linking various lifestyle and medical factors to pregnancy rates
Many fertility databases now contain information on hundreds of thousands of menstrual data, treatments, outcomes, etc.[1] Machine learning analyzes these archives, finding unclear predictors in isolated cases. For example, analysis of the 23andMe genetic database found linked certain genetic variants, such as those affecting BMI, to lower fertility - with a biological chance of getting pregnant each cycle in
Such findings help clinicians better understand each patient’s biology and environment. Equipped with demographic insights, doctors can quickly spot trends and make lifestyle recommendations accordingly - such as dietary changes or weight management for individual carriers with high-risk genes.
By focusing on personalized techniques and the usage of advanced technology, this paradigm shift empowers people to take energetic manipulate in their health, growing a lifestyle of well-being. It emphasizes early intervention and tailored solutions, ultimately reducing healthcare costs and improving overall outcomes. This transformative approach promotes a holistic understanding of health considering physical and psychological well-being to create a healthy and sustainable health care system.
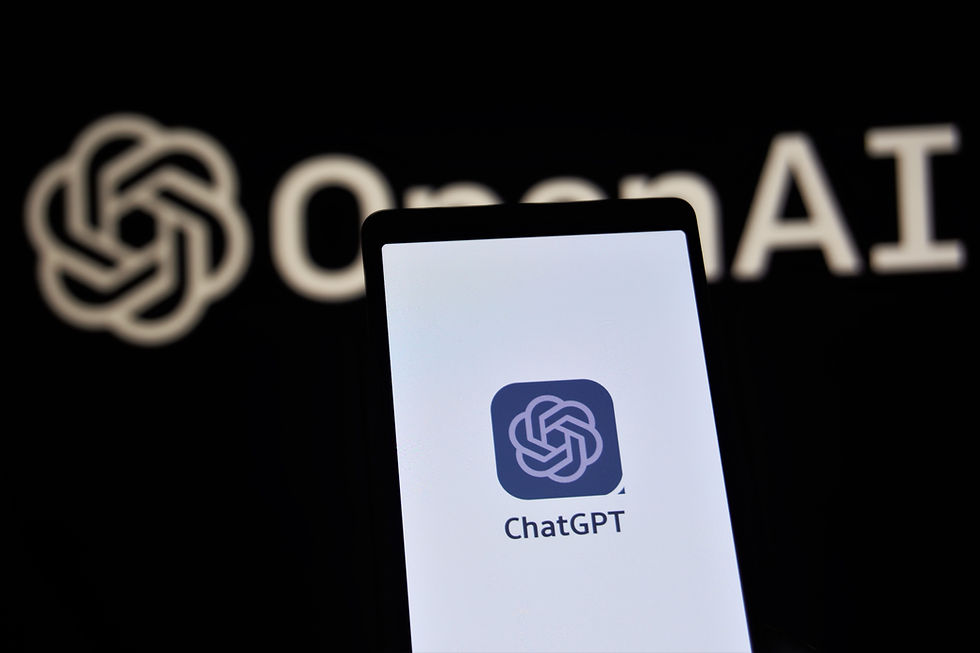
AI Assists in Diagnosis and Treatment Planning
Artificial intelligence also improves diagnostic capabilities. By digitizing millions of medical records describing different symptoms, treatments, and outcomes, AI systems learn to spot subtle patterns indicating particular conditions. Built-in knowledge of the latest research further sharpens their diagnostic skills.[3]
Several startups now offer AI-powered fertility assessment tools. For example, Ava Women's Health uses data from its wearable fertility tracker to train algorithms that can determine ovulation with over 90% accuracy based on various biomarkers like temperature and heart rate. Its AI detects abnormalities like luteal phases that are too short and could signal infertility issues.[4]
Advanced machine learning is also assisting with in vitro fertilization (IVF) treatment planning. By analyzing huge troves of IVF outcomes linked to patient profiles, dosing protocols, embryo characteristics, and more, AI can predict the likeliest treatment path for success on the first attempt.[5] This spares couples from multiple emotionally and financially taxing rounds while boosting the odds of live birth.
Predictive algorithms also explore personalized embryo selection based on morphological scoring and chemical profiles that correlate highly with implantation potential. This data-informed selection process grows more effective with each new profile added. Such tools support clinicians in optimizing care based on individual biology rather than generic protocols.
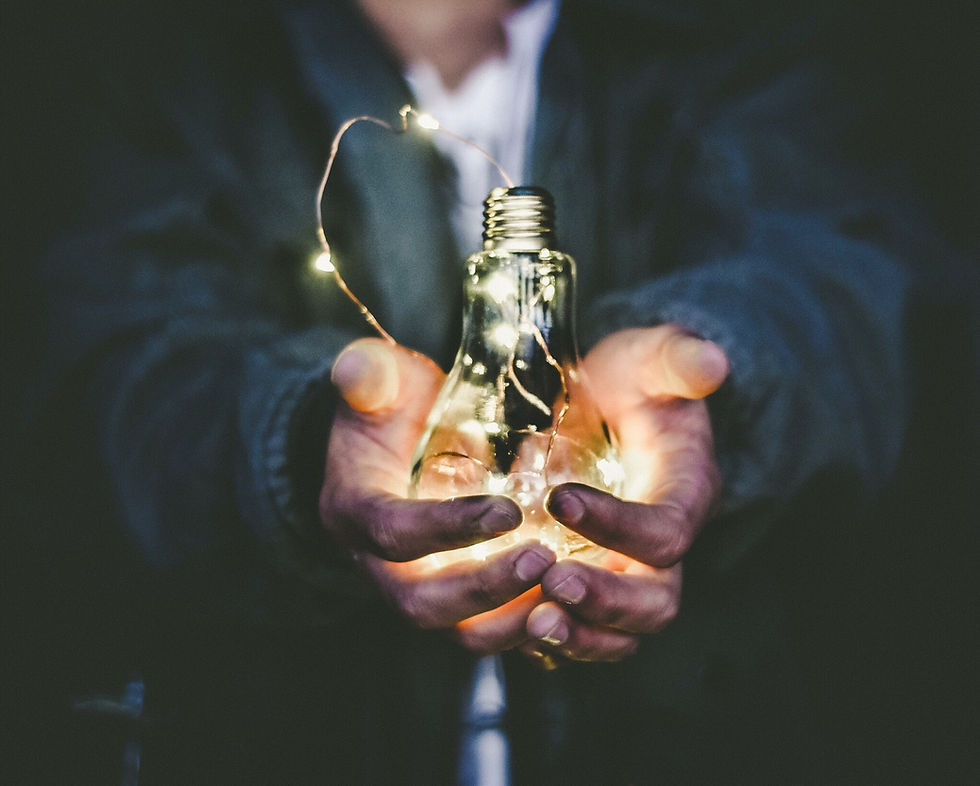
Ethical Considerations as Personal Data Expands
The flow of personal reproductive data certainly raises ethical issues regarding its use in terms of responsibility and consent. Strict privacy and security standards should protect sensitive health information and protect it from risks of rediscovery. Transparency about data application and a process for opting out are also crucial for building trust with patients.
Additionally, genetic and predictive findings require sensitive interpretation and confirmation through follow-up discussions with a medical advisor. While the technology shows compatibility, the primary care physician can weigh the benefits against the risks by taking into account each person’s overall medical and family history. AI alone should never dictate a diagnosis or treatment plan without human oversight and approval.
Overall, as long as privacy is respected and medical professionals thoughtfully guide the next steps, data-fueled innovation empowers individuals and doctors with deeper insight into complex fertility matters. No longer are individuals left wondering alone - new solutions consider all biological influences together to demystify and address underlying challenges driving difficulties in conceiving.[6]
Conclusion
Once a confounding medical mystery, infertility is gradually yielding its secrets to the power of aggregated patient data and advanced analytics. By illuminating correlations across lifestyle, genetics, and environments, these new data-focused approaches support precision diagnosis and customized care for each unique biology and circumstance.
Moving forward, continued collaboration between technology experts, medical researchers, and providers will refine these tools, expanding their positive impact. As norms around data access and sharing evolve responsibly, a future of family planning personalized down to each individual comes into focus - one driven not only by chance alone but also by science, empowerment, and hope.
References:
Papatheodorou, S. I., Alhopuro, P. & Lindfors, K. Leveraging large population-scale data resources to study human fertility and reproductive health. Genome Med 13, 13 (2021).
Li, T., Drinkwater, B. D., Moore, A. Y., Hinsdale, M., Palomino, W., Osborne, J., Cutler, D. J. (2020). An atlas of genetic influences on human life history traits. Genetics, 216(3), 769–780.
Vien, L., Dubé, J., Qian, C., Zhao, L. Artificial Intelligence in Fertility and Reproductive Medicine: Current Applications and Perspectives. Front Med (Lausanne). 2021 Mar 11;8:641116.
Ava Women's Health. Product Founder.
Lee, J, Joo, H, Kim, S, Moon, S. An artificial intelligence-based prediction model for live birth after in vitro fertilization. J Obstet Gynaecol Res. 2022 Feb;48(2):454-461.
Practice Committees of the American Society for Reproductive Medicine and the Society for Assisted Reproductive Technology. The use of predictive mathematical models and biomarkers for selection of embryos for transfer following in vitro fertilization: a committee opinion. Fertil Steril. 2017 Dec;108(6):1240-1244.
Comentários